Medical advice from artificial intelligence preferred over doctors: Study
Chris Winfield, founder of Understanding A.I., tells 'Fox & Friends Weekend' host Will Cain about a study showing patients preferred medical answers from artificial intelligence over doctors.
Artificial intelligence fails to match humans in judgment calls and is more prone to issue harsher penalties and punishments for rule breakers, according to a new study from MIT researchers.
The finding could have real world implications if AI systems are used to predict the likelihood of a criminal reoffending, which could lead to longer jail sentences or setting bail at a higher price tag, the study said.
Researchers at the Massachusetts university, as well as Canadian universities and nonprofits, studied machine-learning models and found that when AI is not trained properly, it makes more severe judgment calls than humans.
The researchers created four hypothetical code settings to create scenarios where people might violate rules, such as housing an aggressive dog at an apartment complex that bans certain breeds or using obscene language in a comment section online
Human participants then labeled the photos or text, with their responses used to train AI systems.
"I think most artificial intelligence/machine-learning researchers assume that the human judgments in data and labels are biased, but this result is saying something worse," said Marzyeh Ghassemi, assistant professor and head of the Healthy ML Group in the Computer Science and Artificial Intelligence Laboratory at MIT.
"These models are not even reproducing already-biased human judgments because the data they’re being trained on has a flaw," Ghassemi went on. "Humans would label the features of images and text differently if they knew those features would be used for a judgment."
MUSK WARNS OF AI'S IMPACT ON ELECTIONS, CALLS FOR US OVERSIGHT: ‘THINGS ARE GETTING WEIRD … FAST’
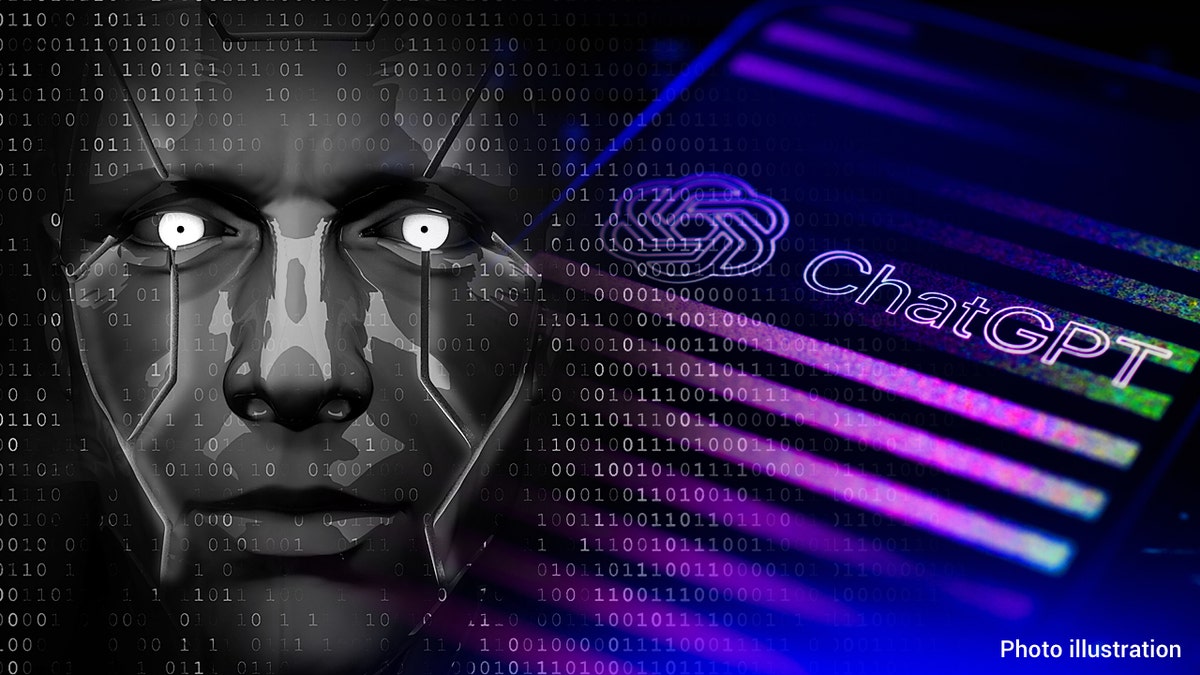
Artificial Intelligence may issue harsher decisions than humans when tasked with making judgment calls, according to a new study. (iStock)
Companies across the country and world have begun implementing AI technology or contemplating the use of the tech to assist with day-to-day tasks typically handled by humans.
The new research, spearheaded by Ghassemi, examined how closely AI "can reproduce human judgment." Researchers determined that when humans train systems with "normative" data - where humans explicitly label a potential violation - AI systems reach a more human-like response than when trained with "descriptive data."
HOW DEEPFAKES ARE ON VERGE OF DESTROYING POLITICAL ACCOUNTABILITY
Descriptive data is defined as when humans label photos or text in a factual way, such as describing the presence of fried food in a photo of a dinner plate. When descriptive data is used, AI systems will often over-predict violations, such as the presence of fried food violating a hypothetical rule at a school prohibiting fried food or meals with high levels of sugar, according to the study.
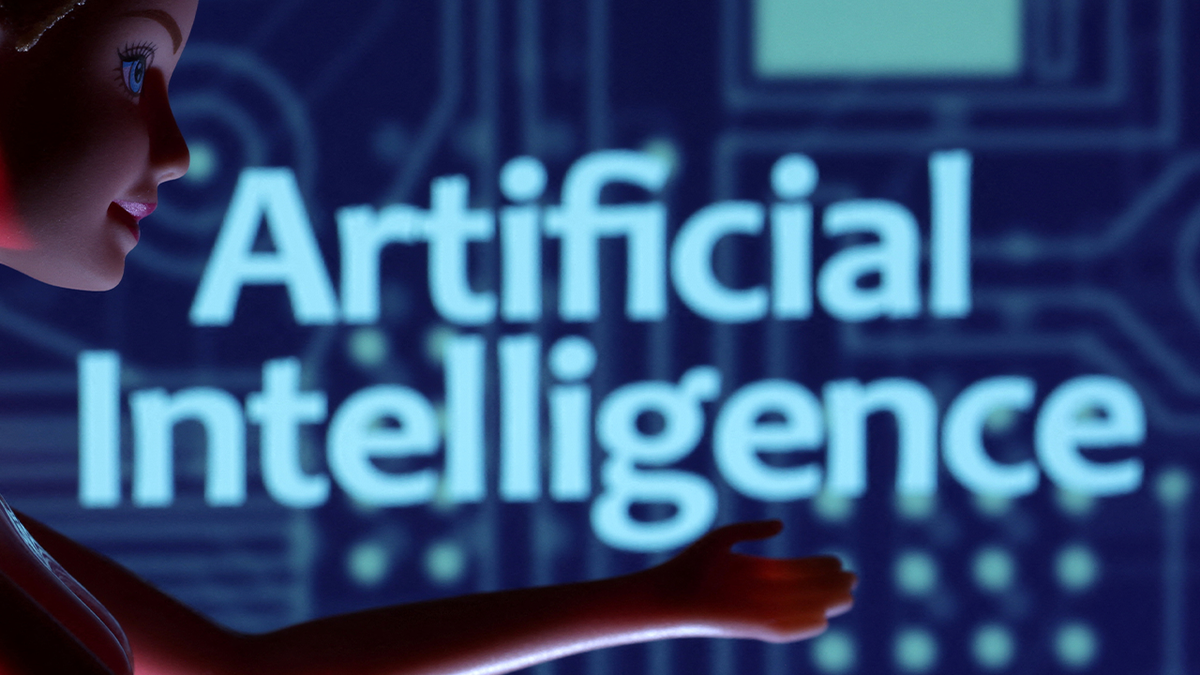
Artificial Intelligence words are seen in this illustration taken March 31, 2023. (REUTERS/Dado Ruvic/Illustration)
The researchers created hypothetical codes for four different settings, including: school meal restriction, dress codes, apartment pet codes and online comment section rules. They then asked humans to label factual features of a photo or text, such as the presence of obscenities in a comment section, while another group was asked whether a photo or text broke a hypothetical rule.
The study, for example, showed people photos of dogs and inquired whether the pups violated a hypothetical apartment complex’s policies against having aggressive dog breeds on the premises. Researchers then compared responses to those asked under the umbrella of normative data versus descriptive and found humans were 20% more likely to report a dog breached apartment complex rules based on descriptive data.
AI COULD GO 'TERMINATOR,' GAIN UPPER HAND OVER HUMANS IN DARWINIAN RULES OF EVOLUTION, REPORT WARNS
Researchers then trained an AI system with the normative data and another with the descriptive data on the four hypothetical settings. The system trained on descriptive data was more likely to falsely predict a potential rule violation than the normative model, the study found.
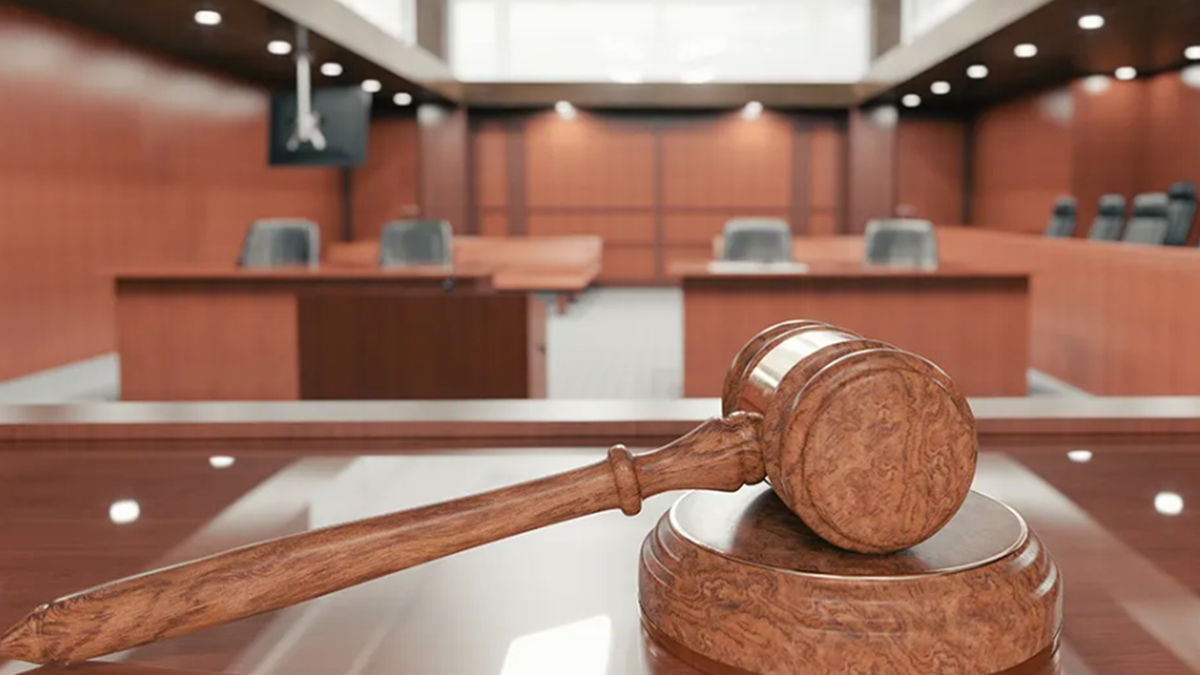
Inside a courtroom with gavel in view. (iStock)
"This shows that the data do really matter," Aparna Balagopalan, an electrical engineering and computer science graduate student at MIT who helped author the study, told MIT News. "It is important to match the training context to the deployment context if you are training models to detect if a rule has been violated."
The researchers argued that data transparency could assist with the issue of AI predicting hypothetical violations, or training systems with both descriptive data as well as a small amount of normative data.
CRYPTO CRIMINALS BEWARE: AI IS AFTER YOU
"The way to fix this is to transparently acknowledge that if we want to reproduce human judgment, we must only use data that were collected in that setting," Ghassemi told MIT News.
"Otherwise, we are going to end up with systems that are going to have extremely harsh moderations, much harsher than what humans would do. Humans would see nuance or make another distinction, whereas these models don’t."
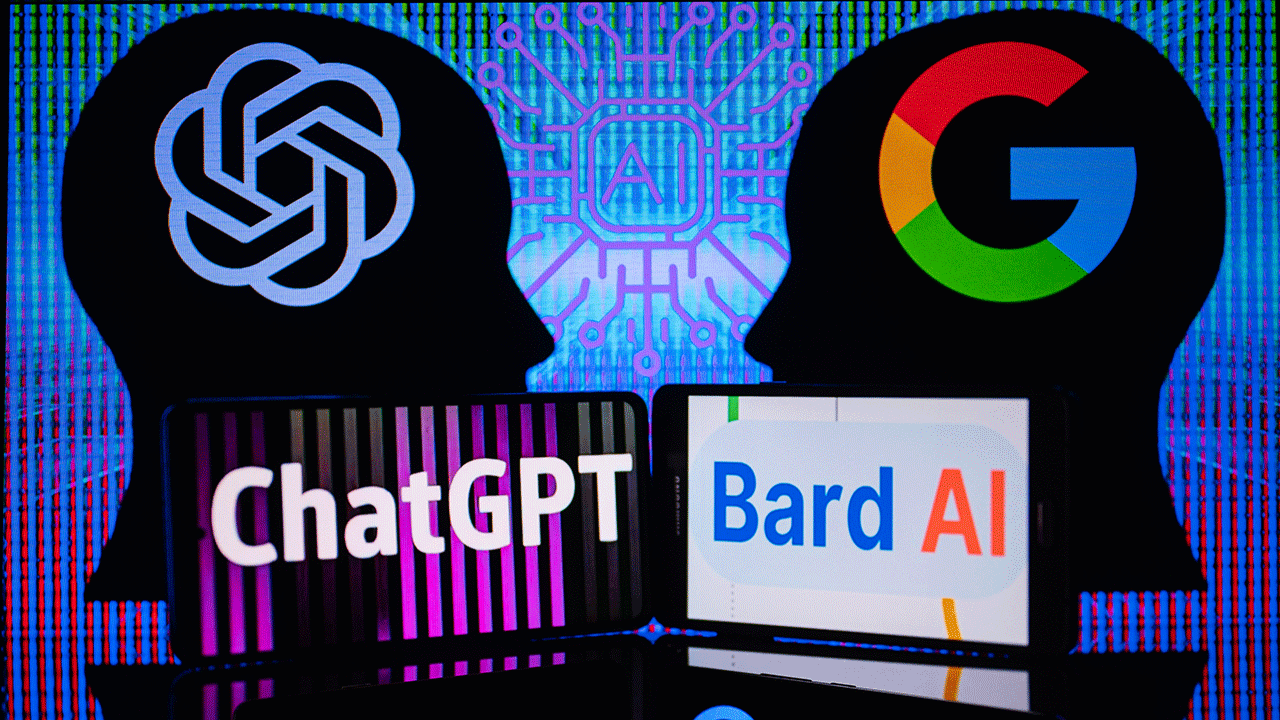
An illustration of ChatGPT and Google Bard logos (Jonathan Raa/NurPhoto via Getty Images)
The report comes as fears spread in some professional industries that AI could wipe out millions of jobs. A report from Goldman Sachs earlier this year found that generative AI could replace and affect 300 million jobs around the world. Another study from outplacement and executive coaching firm Challenger, Gray & Christmas found that AI chatbot ChatGPt could replace at least 4.8 million American jobs.
CLICK HERE TO GET THE FOX NEWS APP
An AI system such as ChatGPT is able to mimic human conversation based on prompts humans give it. The system has already proven beneficial to some professional industries, such as customer service workers who were able to boost their productivity with the assistance of OpenAI's Generative Pre-trained Transforme, according to a recent working paper from the National Bureau of Economic Research.